GenAI vs. Non-GenAI Market Analysis: Understanding the Landscape
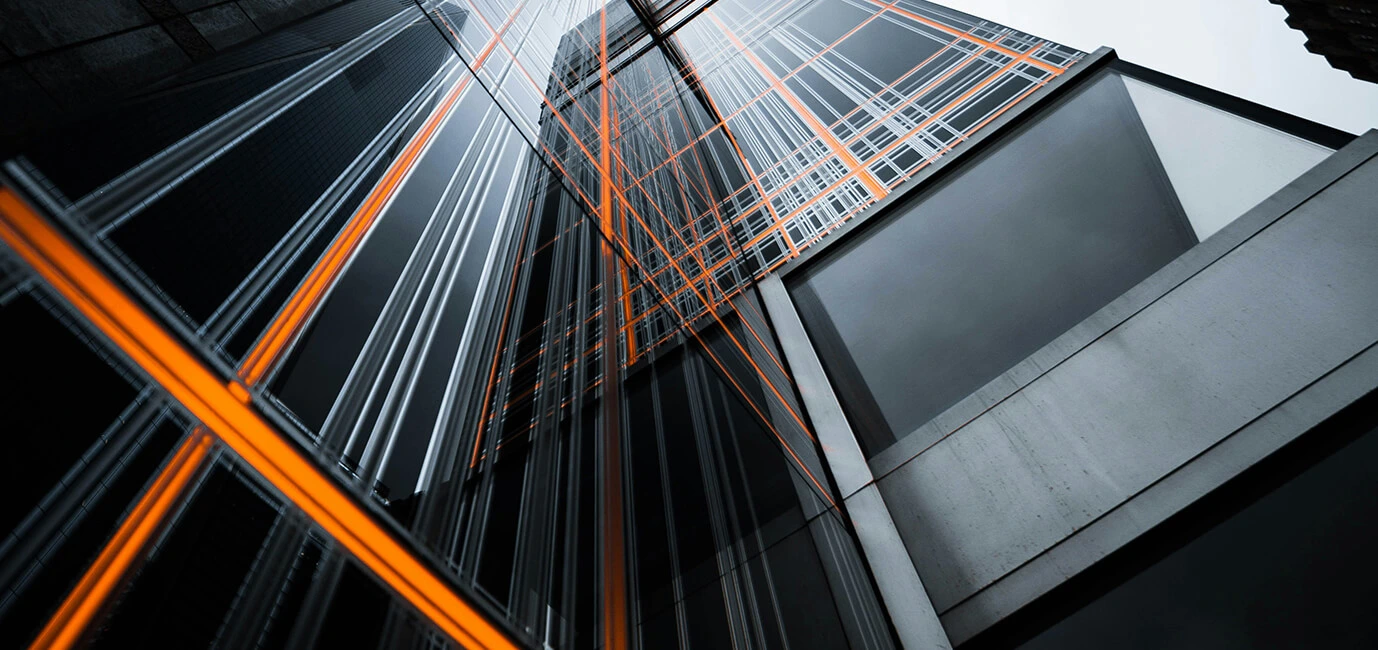
As businesses across industries explore artificial intelligence (AI) to enhance operations, improve customer experiences, and drive innovation, the rise of generative AI (GenAI) has introduced a new paradigm of possibilities. But with all the buzz surrounding GenAI, it’s important to differentiate between GenAI and non-GenAI solutions, especially when it comes to market adoption, infrastructure, and potential pitfalls. This analysis compares GenAI and non-GenAI markets, providing insights into how businesses are spending on AI, the challenges they face, and the key factors that differentiate the two approaches.
Generative AI (GenAI) refers to AI systems that can create new content—whether it's text, images, or even entire business processes—based on existing data. GenAI models, such as OpenAI’s GPT or Google’s Bard, can generate human-like text, create realistic images, or even code software, making them valuable tools for automating complex tasks and producing innovative solutions. These models rely heavily on deep learning techniques to process massive datasets, creating outputs that resemble or improve upon human-generated content.
On the other hand, non-GenAI systems are more focused on decision-making and automation rather than content creation. These systems typically operate within predefined rules and models, handling tasks like predictive analytics, pattern recognition, and data-driven decision-making. While non-GenAI AI systems have been integral in business automation and customer service, GenAI has taken the AI landscape by storm with its creative and adaptive capabilities.
The allocation of resources in GenAI versus non-GenAI initiatives varies significantly. Here's a breakdown of how businesses are investing in GenAI projects, compared to traditional AI solutions:
- Applications and Software: For GenAI, businesses are spending roughly 36% of their budgets on software and applications. This includes both software as a service (SaaS) platforms and custom-built solutions powered by generative models. Non-GenAI spending on software tends to focus more on analytics, machine learning models, and robotic process automation (RPA) tools that streamline operational tasks but do not involve content generation.
- Personnel: Approximately 25% of GenAI investments are directed toward hiring or contracting personnel with the expertise to design, implement, and manage these systems. GenAI requires specialized talent in fields like machine learning, natural language processing, and deep learning, which is a major reason why personnel costs are high. Non-GenAI systems typically require personnel with expertise in data science, software engineering, and systems integration, though the demand for AI specialists in non-GenAI is still significant.
- Infrastructure: GenAI is particularly demanding when it comes to infrastructure, with about 21% of budget allocation going to storage and compute resources. Generating content from large models requires substantial computing power and data storage capacity. For non-GenAI applications, the infrastructure needs are less intense but still substantial, with costs generally associated with maintaining data centers and processing analytical models.
- Outsourcing: For both GenAI and non-GenAI systems, about 18% of spending is allocated to managed services and outsourced solutions. Businesses often rely on external vendors to help scale AI initiatives, particularly when they lack in-house expertise.
While both GenAI and non-GenAI systems have seen widespread adoption, scaling these technologies from pilot projects to full production presents distinct challenges:
- GenAI Scaling: Enterprises often struggle to move GenAI solutions from experimental pilots to full-scale deployments. The complexity of GenAI models and the sheer amount of data needed to fine-tune them for production environments pose significant hurdles. Additionally, ensuring that these systems meet ethical guidelines and governance standards can complicate scaling efforts.
- Non-GenAI Scaling: Non-GenAI systems are generally more straightforward to scale, as they often involve more structured data and predefined workflows. However, organizations still face challenges related to integration, especially when dealing with legacy systems that may not be fully compatible with AI-driven processes.
As more companies adopt GenAI, achieving differentiation in the marketplace becomes a challenge. While GenAI provides innovative capabilities, its widespread availability means that simply using generative models is no longer enough to stand out. Instead, businesses need to focus on unique use cases and efficient scaling of GenAI projects to derive long-term value.
In contrast, non-GenAI solutions, though not as “trendy” as their GenAI counterparts, can still offer significant competitive advantages in terms of operational efficiency, especially in industries that rely on heavy data processing or automation.
One of the most significant challenges in GenAI adoption is governance. Businesses often underestimate the need for strong governance frameworks to ensure ethical use, data privacy, and compliance with regulatory standards. Improved governance and coordination were highlighted as critical factors that enterprises would focus on if they could redo their GenAI initiatives.
For non-GenAI, governance tends to focus more on data quality, security, and operational efficiency. Though the risks are less pronounced than with GenAI, proper governance remains crucial for both types of AI initiatives.
As the market for AI continues to evolve, understanding the distinctions between GenAI and non-GenAI solutions is critical for making informed investment decisions. While GenAI offers groundbreaking capabilities, its scaling challenges, infrastructure demands, and governance issues require careful consideration. Non-GenAI systems, meanwhile, remain valuable for businesses looking to streamline operations and enhance decision-making without the complexity of generative models.
For companies utilizing AI-driven customer service solutions like Pypestream, leveraging the right combination of GenAI and non-GenAI technologies can unlock powerful opportunities for innovation, efficiency, and customer satisfaction.